Reviewing the Pivotal Research “Paper: Scaling Laws for Neural Language Models” and Their Implications
The Most Important Paper for the Modern Transformer Model!
2/5/20243 min read
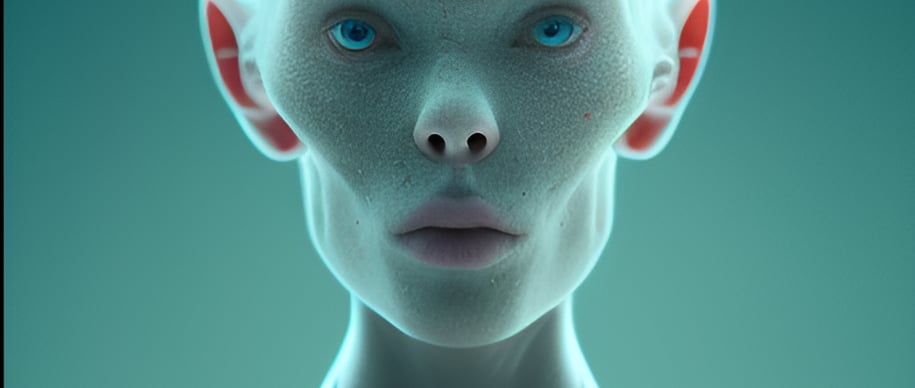
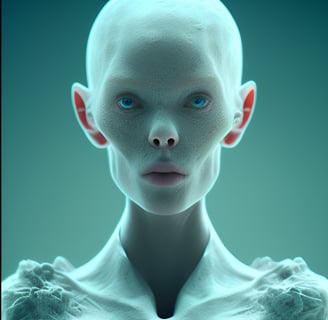
Artificial Intelligence (AI) and Machine Learning (ML) are transforming industries and reshaping the world as we know it. One of the most exciting developments in this field is the rapid progress in Natural Language Processing (NLP), which enables machines to understand, generate, and respond to human language. A recent paper titled "Scaling Laws for Neural Language Models" has shed new light on the potential of NLP and its implications for businesses and professionals. In this article, we will delve into the findings of this groundbreaking research and explore its significance for the future of AI.
The Power of Scaling Laws
The paper, authored by a team of researchers from Google Brain, OpenAI, and the University of California, Berkeley, presents empirical scaling laws for language model performance based on the cross-entropy loss. These scaling laws reveal that the loss scales as a power-law with model size, dataset size, and the amount of compute used for training, with some trends spanning more than seven orders of magnitude.
This discovery has profound implications for the development of AI and ML systems. By understanding these scaling laws, we can determine the optimal allocation of a fixed compute budget, allowing us to build more efficient and powerful models. The researchers found that larger models are significantly more sample-efficient, meaning that they can learn more from a given amount of data. This finding suggests that optimally compute-efficient training involves training very large models on a relatively modest amount of data and stopping significantly before convergence.
The Importance of Model Size and Compute
One of the most striking findings of the paper is the minimal effect of architectural details such as network width or depth on model performance. This suggests that the focus should be on increasing model size and compute, rather than tweaking architectural parameters. The researchers found that a tenfold increase in model size leads to a significant improvement in performance, while a tenfold increase in dataset size or compute results in a smaller, but still substantial, improvement.
This emphasis on model size and compute has important implications for businesses and professionals looking to leverage AI and ML. It suggests that investing in larger models and more powerful compute resources will yield significant returns in terms of improved performance and efficiency. Moreover, it highlights the importance of collaboration and resource sharing in the development of AI and ML systems, as the costs associated with building and training large models can be prohibitive for individual organizations.
The Future of AI: A Brave New World
The findings of the "Scaling Laws for Neural Language Models" paper point to a future in which AI and ML systems are more powerful, efficient, and capable than ever before. As model sizes and compute resources continue to grow, we can expect to see rapid progress in NLP and other areas of AI and ML. This progress will have far-reaching implications for industries ranging from healthcare and finance to transportation and entertainment.
For businesses and professionals, the key to success in this brave new world will be staying ahead of the curve and leveraging the latest advances in AI and ML. This means investing in larger models and more powerful compute resources, as well as developing the skills and expertise needed to harness the full potential of these technologies. It also means staying informed about the latest research and developments in the field, and being prepared to adapt and evolve in response to new challenges and opportunities.
Conclusion
The "Scaling Laws for Neural Language Models" paper is a landmark study that has shed new light on the potential of AI and ML. By understanding the empirical scaling laws for language model performance, we can build more efficient and powerful models that can transform industries and reshape the world. As businesses and professionals, it is our responsibility to stay informed, invest in the right resources, and develop the skills and expertise needed to harness the full potential of these technologies. The future of AI is bright, and it is up to us to seize the opportunities it presents.
Edited and written by David J Ritchie